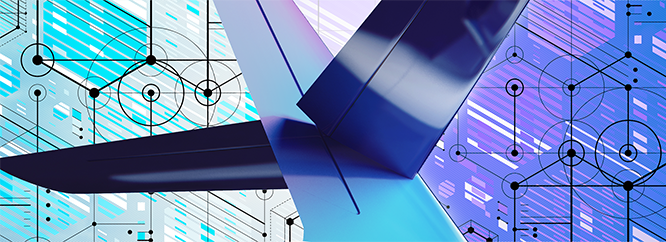
Volume
33
Issue
2
Key words
machine learning, unsupervised learning, integrated systems, theory of polymorphic learning, flight data, garmin g1000
Abstract
Increased availability of data and computing power has allowed organizations to apply machine learning techniques to various fleet monitoring activities. Additionally, our ability to acquire aircraft data has increased due to the miniaturization of small form factor computing machines. Aircraft data collection processes contain many data features in the form of multivariate time series (continuous, discrete, categorical, etc.) which can be used to train machine learning models. Yet, three major challenges still face many flight organizations: 1) integration and automation of data collection frameworks, 2) data cleanup and preparation, and 3) developing an embedded machine learning framework. Data cleanup and preparation have been a well-known challenge since database systems were first invented. While integration and automation of data collection efforts within many organizations is quite mature, there are special challenges for flight-based organizations (i.e., the automatic and efficient transmission of aircraft flight data to centralized analytical data processing systems). Furthermore, this creates additional constraints for the operationalization of embedded machine learning methods for classical tasks such as classification and prediction; and magnifying design challenges for the more novel ‘prescriptive-based’ architectures. Our research is focused on the application of a design pattern for a) the integration and automation of data collection and b) an organizationally embedded ensemble machine learning method.
Scholarly Commons Citation
Pritchard, M. J.,
Walden, A. T.,
& Thomas, P. J.
(2024).
Integrated Organizational Machine Learning for Aviation Flight Data.
Journal of Aviation/Aerospace Education & Research, 33(2).
DOI: https://doi.org/10.58940/2329-258X.2000
Included in
Management Information Systems Commons, Systems Engineering and Multidisciplinary Design Optimization Commons, Systems Science Commons