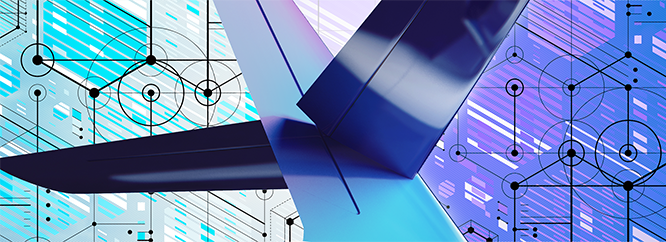
Volume
33
Issue
4
Key words
Data Analytics, Machine Learning, Explainable Artificial Intelligence (XAI), Aviation Safety, Pilot Training, Pilot Schools, FAA Rule-Making, Aviation Incidents
Abstract
Recent pilot shortages have brought pilot training into focus as the industry attempts to rectify a compounding problem. The FAA has implemented some recent rule-making regarding pilot training that has left the General Aviation community questioning the motive or justification behind the rules. FAA incident data are inconsistent, specifically with aviation activity in general; flight activity that does not result in an accident or incident is not recorded for analysis. Despite the present shortcomings in the AIDS data, applying Machine Learning techniques make it possible to predict what characteristics mitigate aircraft damage during an incident (AUC=0.913, Accuracy=97%, Recall=84%, Precision=89%). Machine Learning analysis of incident data may assist in evidence-based rule-making for pilot training. Such rule-making is more likely to impact aviation safety positively and resonate positively within the aviation community. The results highlight the importance of taking immediate steps to improve database quality through improved data governance.
Scholarly Commons Citation
Zierman, R.,
& Cankaya, B.
(2024).
Identifying Aircraft Damage Mitigating Factors with Explainable Artificial Intelligence (XAI): An Evidence-Based Approach to Rule-Making for Pilot Training Schools.
Journal of Aviation/Aerospace Education & Research, 33(4).
DOI: https://doi.org/10.58940/2329-258X.2029