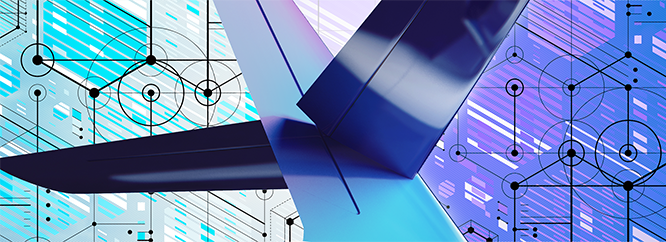
Volume
33
Issue
4
Key words
unmanned aerial vehicle, UAV, safety distance, reinforcement learning, deep deterministic policy gradient, flight safety, airspace efficiency, UAV fleet operation
Abstract
In the field of aviation, safety is a critical cornerstone, and the operation of Unmanned Aerial Vehicle (UAV) systems is deeply connected with this principle. A thorough analysis and rigorous simulation and testing of aircraft systems are essential to avoid severe safety hazards. This paper delves into the safety issue in UAV operations, specifically regarding maintaining minimum safety distances under fluctuating wind conditions. The study introduces a novel solution based on a Deep Deterministic Policy Gradient (DDPG) model, a reinforcement learning method. The DDPG model was trained using a simulated environment created through the Gazebo simulator, with values for wind and gust conditions derived from historical records at the KLAF airport at Purdue University. The model's performance was evaluated regarding maintaining safe distances under these conditions. The results indicate that the DDPG model can accurately predict safety distances with relatively low error rates when predicting under different weather conditions. The findings significantly contribute to UAV safety operations, suggesting the potential future utilization of reinforcement learning methods to study enhancing airspace efficiency and obstruction avoidance in UAVs.
Scholarly Commons Citation
Xu, X.,
& Sun, J.
(2024).
A New Trajectory in UAV Safety: Leveraging Reinforcement Learning for Distance Maintenance Under Wind Variations.
Journal of Aviation/Aerospace Education & Research, 33(4).
DOI: https://doi.org/10.58940/2329-258X.2045
Included in
Management and Operations Commons, Multi-Vehicle Systems and Air Traffic Control Commons, Navigation, Guidance, Control and Dynamics Commons