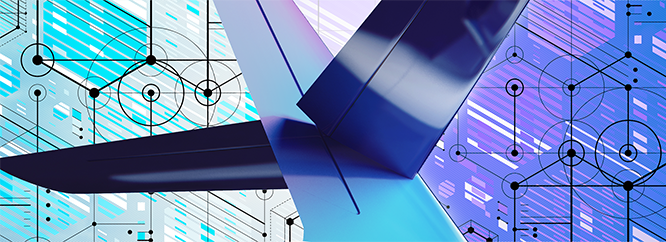
Volume
33
Issue
4
Key words
automatic speech recognition, aviation maintenance logbooks, domain adaptation
Abstract
With timeliness and efficiency being critical in the aviation maintenance industry, the need has been growing for smart technological solutions that optimize and streamline the different underlying tasks (Bergkvist & Sabbagh, 2021). One such task is the technical documentation of the performed maintenance operations (Chandola et al., 2022). Instead of manual documentation, voice tools that transcribe spoken logbook entries allow technicians to document their work right away in a hands-free and time efficient manner. However, an accurate automatic speech recognition (ASR) model requires large training corpora (Siyaev & Jo, 2021a), which are lacking in the domain of aviation maintenance. In addition, ASR models which are trained on huge corpora in standard English perform poorly in such a technical domain with non-standard terminology (Siyaev & Jo, 2021a, 2021b). Hence, this study investigates the extent to which fine-tuning an ASR model, pre-trained on standard English corpora, on limited in-domain data improves its recognition performance in the technical domain of aviation maintenance. We present a case study on one such pre-trained ASR model, wav2vec 2.0 (Baevski et al., 2020). Results showed that fine-tuning the model on a limited anonymized dataset of maintenance logbook entries significantly reduced its error rates when tested on not only an anonymized in-domain dataset, but also a non-anonymized one. This suggests that any available aviation maintenance logbooks, even if anonymized for privacy, can be used to fine-tune general-purpose ASR models and enhance their in-domain performance. Lastly, an analysis on the influence of voice characteristics on model performance stressed the need for balanced datasets representative of the population of aviation maintenance technicians.
Scholarly Commons Citation
Amin, N.,
Yother, T. L.,
& Rayz, J.
(2024).
Low-Resource Automatic Speech Recognition Domain Adaptation – A Case-Study in Aviation Maintenance.
Journal of Aviation/Aerospace Education & Research, 33(4).
DOI: https://doi.org/10.58940/2329-258X.2052