Submitting Campus
Daytona Beach
Student Status
Graduate
Class
Graduate Student Works
Advisor Name
Dr. Riccardo Bevilacqua
Abstract/Description
To continue space operations with the increasing space debris, accurate characterization of fragment fly-out properties from hypervelocity impacts is essential. However, with limited realistic experimentation and the need for data, available static arena test data, collected utilizing a novel stereoscopic imaging technique, is the primary dataset for this paper. This research leverages machine learning methodologies to predict fragmentation characteristics using combined data from this imaging technique and simulations, produced considering dynamic impact conditions. Gaussian mixture models (GMMs), fit via expectation maximization (EM), are used to model fragment track intersections on a defined surface of intersection. After modeling the fragment distributions, k-nearest neighbor (K-NN) regressors are used to predict the desired characteristics. Using Monte Carlo simulations, the K-NN regression is shown to predict the distributions for both the total number of fragments intersecting a given surface, as well as the expected total fragment velocity and mass associated with that surface. This information can then be used to estimate the kinetic energy of the particle to classify the particle and avoid debris collisions.
Document Type
Article
Publication/Presentation Date
8-2023
Publication Title
Acta Astronautica
DOI
https://doi.org/10.1016/j.actaastro.2023.04.036
Publisher
Elsevier
Scholarly Commons Citation
Katharine E. Larsen, Riccardo Bevilacqua, Omkar S. Mulekar, Elisabetta L. Jerome, Thomas J. Hatch-Aguilard, “Predicting Dynamic Fragmentation Characteristics from High-Impact Energy Events Utilizing Terrestrial Static Arena Test Data and Machine Learning”, Acta Astronautica, Volume 209, August 2023, Pages 67-81. https://doi.org/10.1016/j.actaastro.2023.04.036
Grant or Award Name
U.S. Air Force Office of Scientific Research (award number FA9550-20-1-0200)
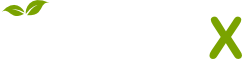
- Citations
- Citation Indexes: 3
- Usage
- Downloads: 99
- Abstract Views: 13
- Captures
- Readers: 14
- Mentions
- News Mentions: 1